It’s hard to move these days for the number of AI-powered chatbots: including the now-ubiquitous ChatGPT. The possibilities are endless, from replying to emails to looking for recipe inspiration to end your four-day pasta streak. But the algorithms underpinning these bots can also classify information. Take a movie review, for instance: ChatGPT can distinguish between positive or negative feedback.
In my PhD research, I’m using the same algorithmic architecture that underpins these bots—also known as large language models, or LLMs—to classify decades of environmental data. Specifically, I’m asking: given historic and forecasted physical conditions in the ocean, which locations would get a positive review for their long-term ability to support coral and other reef-building organisms?
The ongoing devastation of the world’s reefs, which support millions of livelihoods and more than a quarter of the oceans’ biodiversity, has come to symbolize climate change. My research looks at the future for coral reefs through a realistic yet optimistic lens, by pinpointing regions where corals are most likely to survive under climate change, thereby guiding reef conservation and restoration efforts.
Many studies forecast the outlook for reefs using only limited environmental data, such as information on sea surface temperature. They also tend to simplify how ocean conditions change with time by using annual ranges and averages, therefore not capturing the complexity of ocean and coral interactions fully.
Using AI or machine learning allows us to feed as much environmental information as possible into our models forecasts, in turn making their predictions more accurate. These models can process raw time series of many different conditions such as salinity, nutrient concentrations, and underwater currents. Models can also pick out important trends and details from the data, in the same way that they can learn the complex patterns of human language and logic. Given that reef-building corals are complex organisms that respond to environmental triggers with the same level of nuance as complex linguistics, representing these interactions realistically is important.
My initial results (presented at the European Coral Reef Symposium this summer) look promising. A machine learning model can recreate (see maps below) the distribution of reefs that we can today see around Australia, given information about the water depth, warmth, salinity, and circulation over the past 150 years.
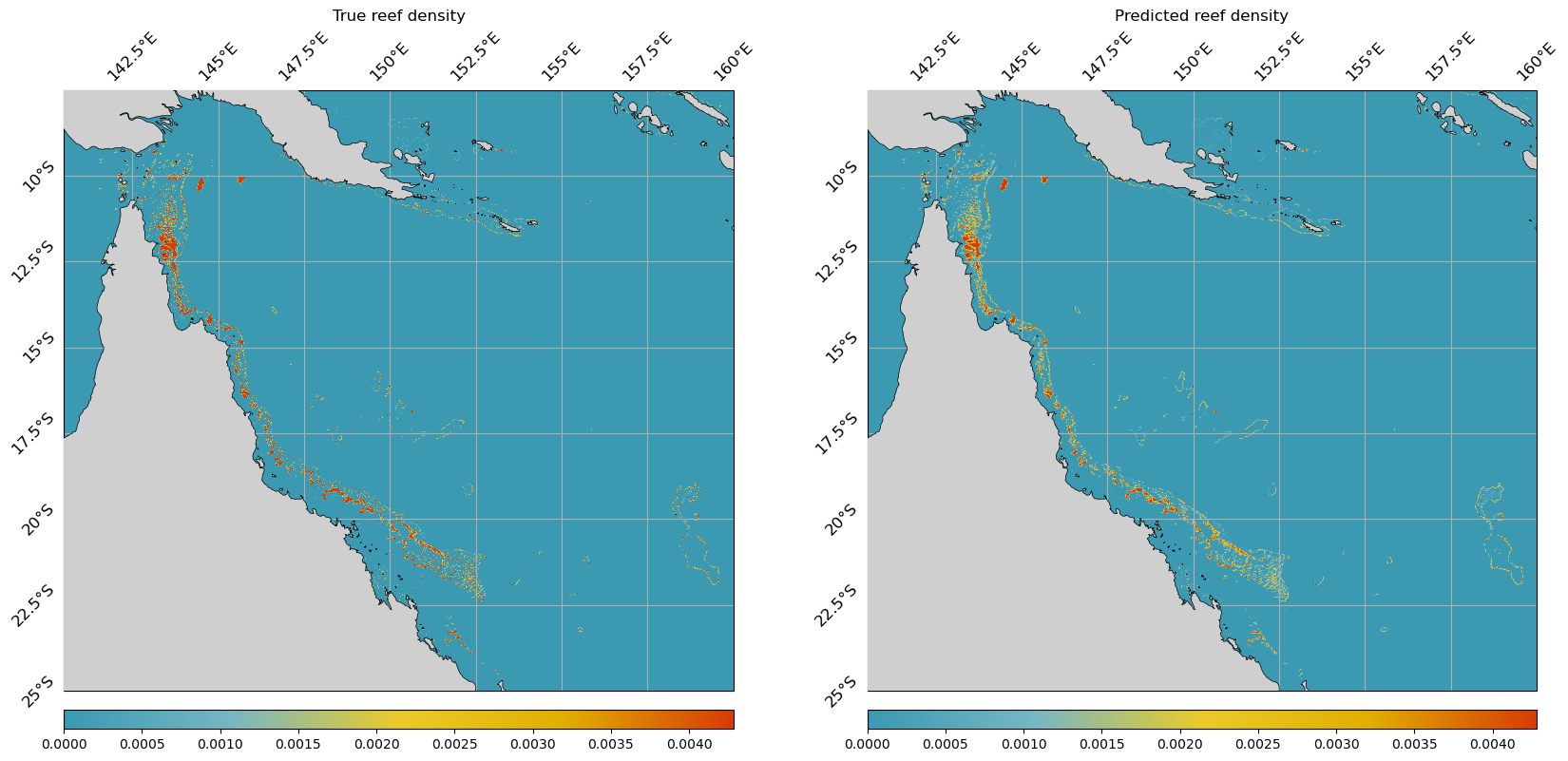
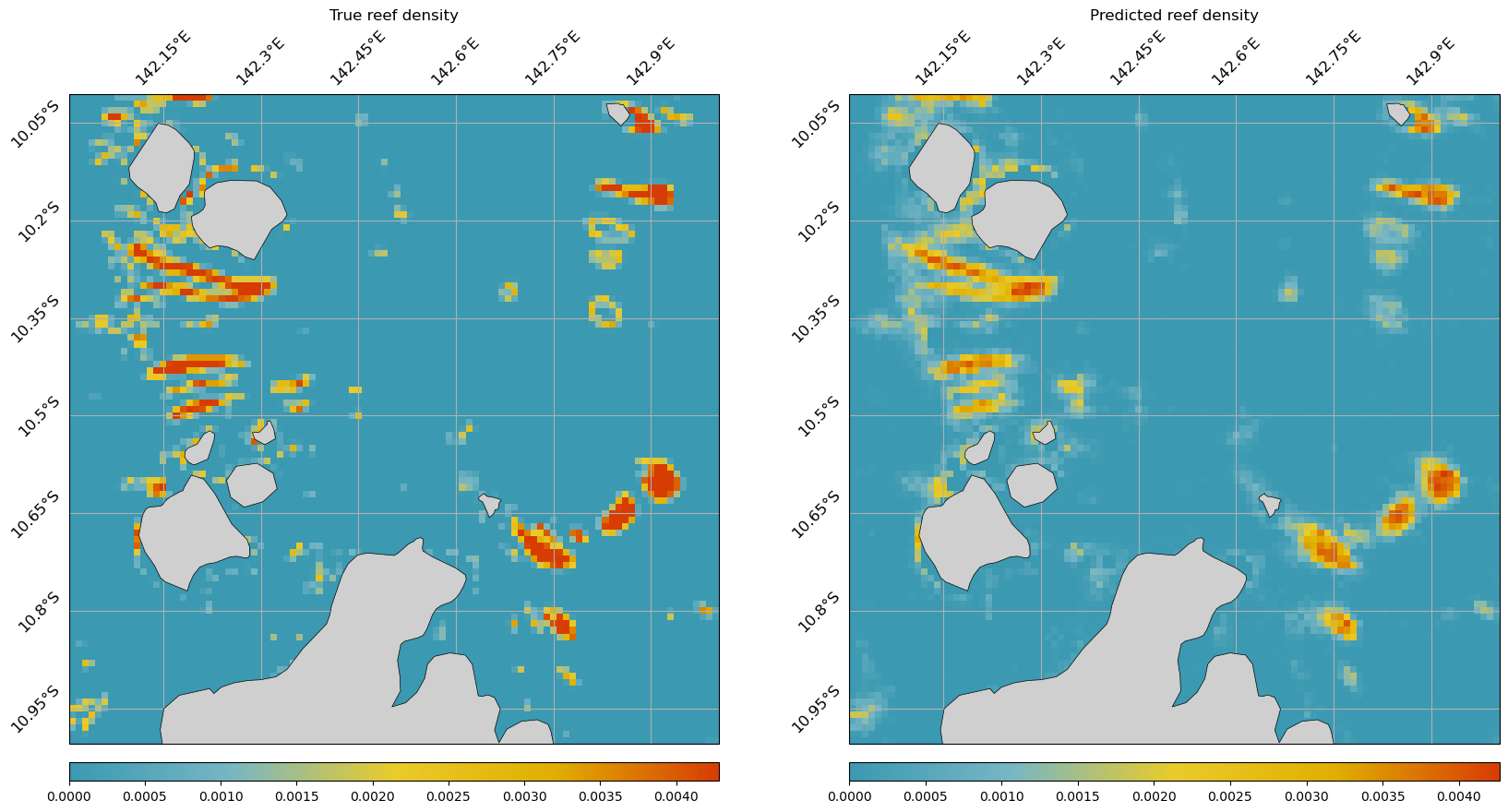
How do we extract all of this complex environmental data? We turn to the Coupled Model Intercomparison Project (that’s CMIP to anyone in a hurry), a collection of numerical atmospheric and oceanographic models created by academic and commercial institutions from around the world. These models underpin the IPCC reports and represent our best guess of historic and future climates.
Climate models divide the planet into grid squares and simulate weather patterns and other climate processes within each block. But even the best climate models can only get down to a spatial resolution of around 25km, which is massive compared to a reef system, let alone reef-building organisms! I’m working to improve this spatial resolution, effectively giving our climate models new glasses to see the ocean shallows in more detail.
Of course, people are trained – and rightly so – to hear warning bells any time AI is touted as the solution to a difficult problem. It’s vital for any application of AI to be well-judged and rigorously tested before it’s ever allowed to see the light of day (or in my case, taste the ocean). My work is just a first step to help guide conservation: it will need to be supplemented with local knowledge on a region-by-region basis before any interventions go ahead.
Orlando Timmerman is a PhD student using machine learning to understand where corals like to live. Orlando is working with Cambridge Earth Science Dr Oscar Branson and his PhD is hosted by the AI4ER (Application of Artificial Intelligence to the study of Environmental Risks) Centre for Doctoral Training programme.
Feature image: Orlando surveying reef ecology in Bermuda, credit: Nicole Burt.